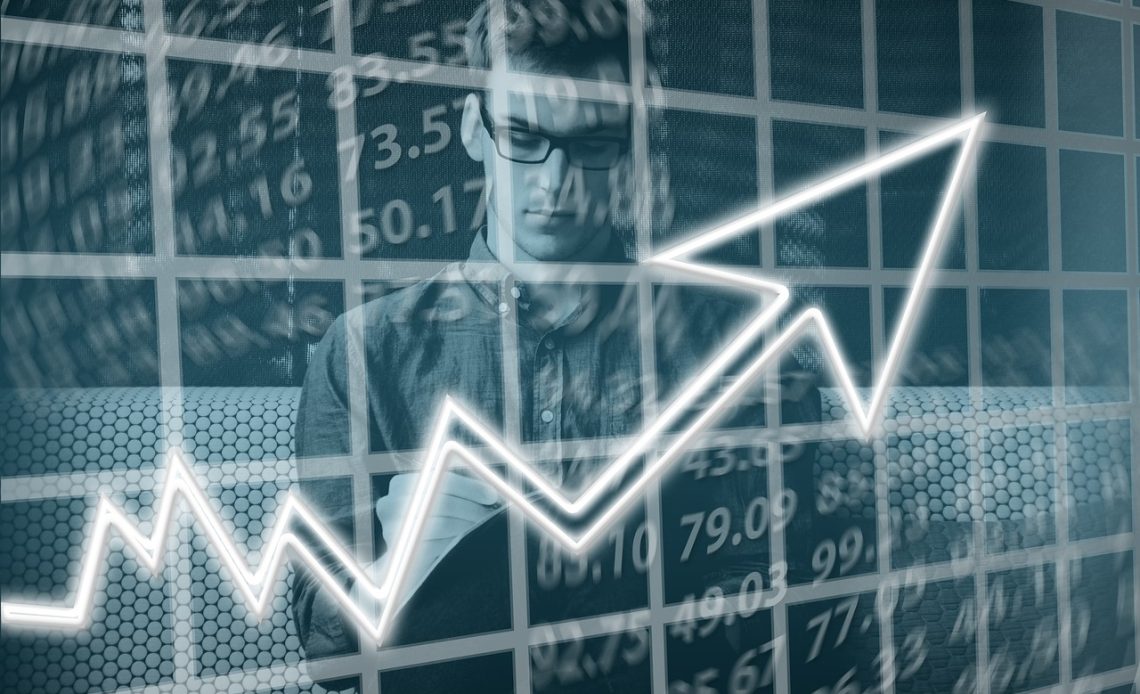
Today, the business world is characterized by rapid change, with companies needing to be more agile, informed, and adaptive than ever before. A critical enabler in this new landscape is the application of Data Analytics and Automation. These two pillars serve to transform the vast seas of data into actionable insights and drive business operations efficiently.
Table of Contents
The Intersection of Data Analytics and Automation
When combined, Data Analytics and Automation create a powerful toolkit for businesses. Here’s why:
- Strategic Decision Making: Data Analytics enables organizations to make decisions anchored in real-time insights. By understanding the intricate differences between Quantitative vs Qualitative Data, organizations can derive richer, more nuanced insights.
- Enhanced Operational Efficiency: Automation streamlines and standardizes repetitive tasks. This reduction in manual labor reduces the likelihood of human errors. This principle is evident in the systematic Data Analysis Process, where automation can assist in consistent data cleaning and processing.
- Agility in Business Operations: Leveraging Data Analytics and Automation allows organizations to respond promptly to market changes, ensuring they remain competitive.
- Improved Customer Experiences: Analytical insights can furnish businesses with deep customer understanding, and automation can subsequently be used to tailor personalized customer interactions, boosting satisfaction levels.
- Cost Management: Efficient processes facilitated by automation can lead to significant cost savings, while analytics can highlight areas for financial optimization.
- Risk Mitigation: Data Analytics identifies potential operational risks, allowing businesses to devise strategies, and automation ensures swift countermeasures.
Reputed business journals, such as McKinsey & Company, have emphasized the transformative capabilities of integrating Data Analytics and Automation for business augmentation.
Analytics vs. Automation: Key Differences
Criteria | Analytics | Automation |
---|---|---|
Definition | Refers to the systematic analysis of data to derive insights. | Refers to the use of technology to perform tasks with minimal human intervention. |
Primary Objective | Informed decision-making, Predictive analysis, and Enhanced understanding of trends. | To streamline processes, increase efficiency, and reduce manual efforts. |
Tools & Technologies | Data visualization tools (e.g., Tableau), Statistical software (e.g., R, Python). | Cost savings, Faster processing times, and Consistency in tasks. |
Key Benefits | Market research, Financial forecasting, and Customer behavior analysis. | Repetitive tasks, Data migration, and Process optimization. |
Applications | Robotic Process Automation (RPA) tools and scripting languages. | Process mapping, Scripting, and Systems integration. |
Skills Required | Statistical analysis, Data visualization, Critical thinking. | Process mapping, Scripting, Systems integration. |
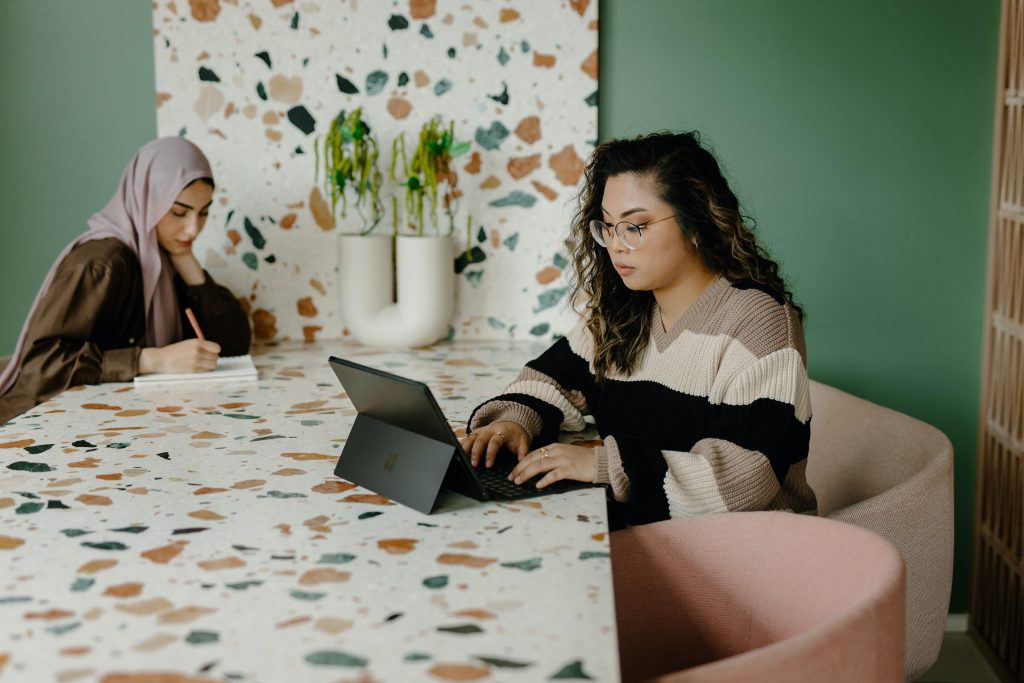
Valuing Expertise: A Look at Salaries
With the increasing significance of Data Analytics and Automation, professionals adept in these domains are highly sought after. Here’s an overview of the average annual salaries in some leading countries:
Country | Data Analysts | Automation Engineers |
---|---|---|
USA | $85,000 | $95,000 |
UK | £45,000 | £50,000 |
Canada | CAD 75,000 | CAD 82,000 |
Germany | €50,000 | €58,000 |
Australia | AUD 78,000 | AUD 85,000 |
For a deeper dive into specific roles within the Data Analytics realm, refer to Data Analyst Salary in NY.
Future Landscape of Data Analytics and Automation
The potential of Data Analytics and Automation extends beyond the immediate. These domains are continuously evolving, driven by advancements in technology and growing data generation. With the rise of the Internet of Things (IoT), 5G, and increased cloud adoption, the future promises an even more integrated, data-driven business ecosystem.
In Summary
The convergence of Data Analytics and Automation serves as a linchpin for contemporary businesses. By turning data into knowledge and then action, businesses can stay ahead of the curve. Whether considering the dynamics of Data Science vs. Data Engineering or mulling over the merits of Data Analytics vs. Software Development, the emphasis should invariably be on leveraging data for optimum business functionality.
FAQ’s
Q1. Is automation part of data analytics?
While automation and data analytics are distinct concepts, they often intersect in modern business operations. Automation in data analytics pertains to streamlining repetitive data processes, such as data collection, cleaning, and processing. By automating certain analytical tasks, businesses can achieve greater efficiency and ensure consistent results.
Q2. Why is automation important in data analytics?
Automation plays a pivotal role in data analytics for several reasons:
- Efficiency: Automated processes can handle large datasets more swiftly than manual operations.
- Consistency: Automation ensures uniformity in data processing, reducing the risk of inconsistencies.
- Scalability: As businesses grow, the amount of data they handle often increases.
- Cost-Effectiveness: In the long run, automating routine data tasks can lead to significant cost savings by reducing manual labor hours.
- Timely Insights: Automated analytics can provide real-time insights, allowing businesses to make more informed decisions promptly.
Q3. How does data science help automation?
Data science complements automation in several ways:
- Predictive Analysis: Data science can predict trends and patterns, enabling automation tools to make proactive decisions.
- Optimization: Algorithms developed through data science can optimize automated processes, ensuring they operate at peak efficiency.
- Personalization: Data science can analyze user behaviors and preferences, guiding automation tools to deliver personalized user experiences.
- Anomaly Detection: Data science models can identify anomalies or irregularities in processes, allowing automated systems to address or flag them.
- Decision Automation: Advanced data science models, especially those using machine learning, can drive decision automation, where systems make decisions without human intervention based on analyzed data.