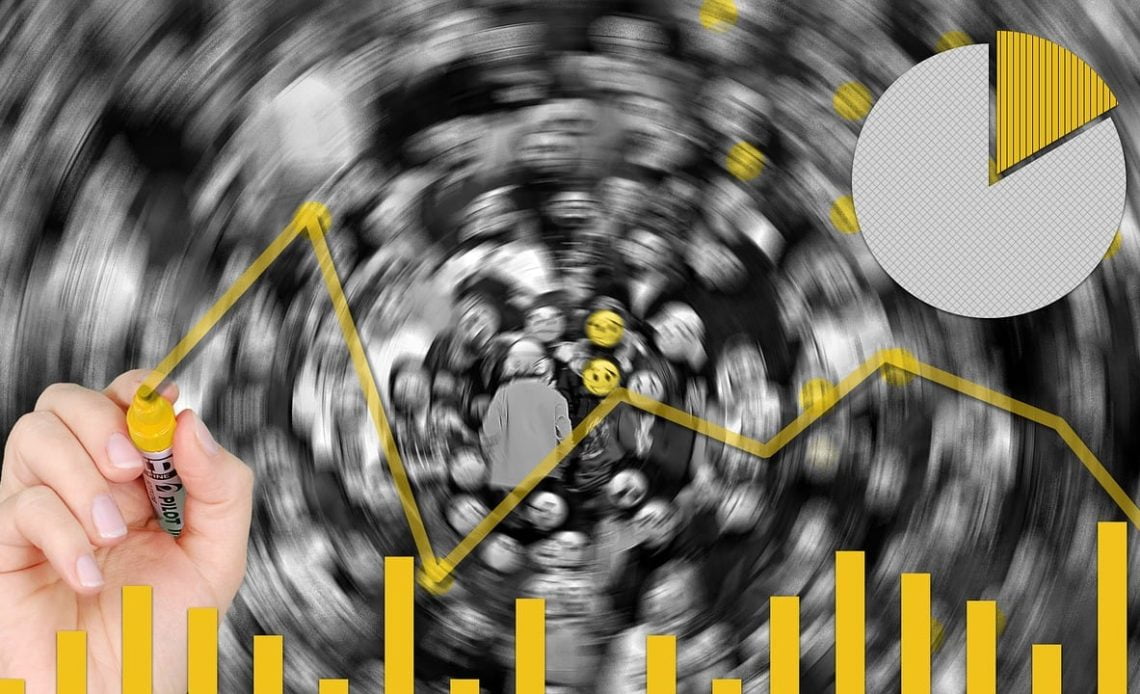
In the digital age, data is often considered the ‘new oil’. It fuels business strategies, informs decision-making, and can lead to breakthrough insights. As the volume of data available grows, understanding how to analyze it becomes even more crucial. This article sheds light on the seven most valuable data analysis methods and techniques, explores the veracity of common assertions regarding data analysis, introduces top tools in the sector, and offers a glimpse into the compensation landscape for data analysts across critical countries.
Table of Contents
1. Descriptive Analysis
This is the starting point for most data examination tasks. Descriptive analysis summarizes raw data and makes it interpretable. It uses measures of central tendency (like mean, median, and mode) and dispersion (like range and standard deviation) to offer a snapshot of data features.
2. Diagnostic Analysis
Once we have a summary of the descriptive analysis, we might ask, “Why did this happen?” Diagnostic analysis delves deeper, seeking to uncover the causes of observed outcomes. It often employs techniques like drill-down, data discovery, and correlations.
3. Predictive Analysis
Predictive analysis forecasts future trends by employing statistical algorithms and machine learning techniques. While it can’t predict outcomes completely, it can provide a probabilistic estimate of upcoming events.
4. Prescriptive Analysis
Going beyond predictive analysis, prescriptive analysis not only forecasts but also suggests a specific course of action. It leverages optimization and simulation algorithms to guide decision-making processes.
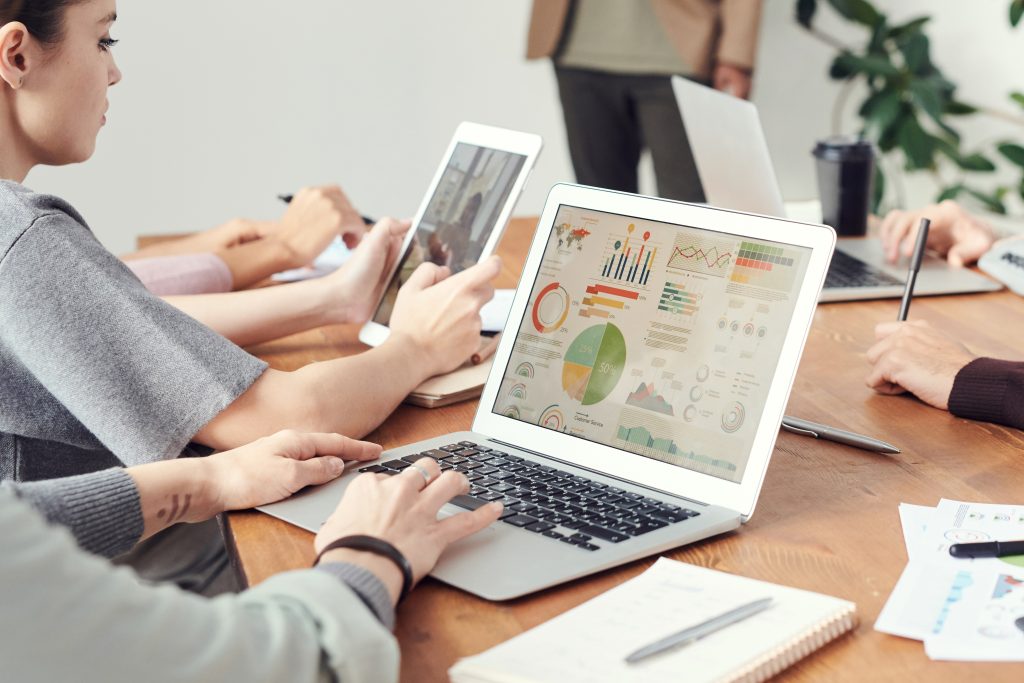
5. Exploratory Data Analysis (EDA)
EDA is an approach that employs visual methods to spot patterns, relationships, anomalies, or any striking characteristics within data. It’s precious during preliminary research stages, where hypotheses are yet to be formed.
6. Inferential Analysis
This technique draws inferences about populations based on sample data. It uses statistical models to deduce or infer trends about a larger population, helping in hypothesis testing.
7. Causal Analysis
Also known as causal inference, this method determines the cause-and-effect relationship between variables. It’s particularly useful in experimental models where certain conditions are controlled and manipulated.
Which of the following is true regarding data analysis?
When navigating the world of data analysis, various assertions arise. To set the record straight:
- Data analysis is purely quantitative: False. Qualitative data analysis involving non-numerical data, like text or images, is vital in various sectors.
- Larger data always yields better results: False. Data quality, relevance, and cleanliness matter more than sheer volume.
- All data analysis requires machine learning: False. While ML is a powerful tool, many analysis tasks are achieved through simpler, classical statistical methods.
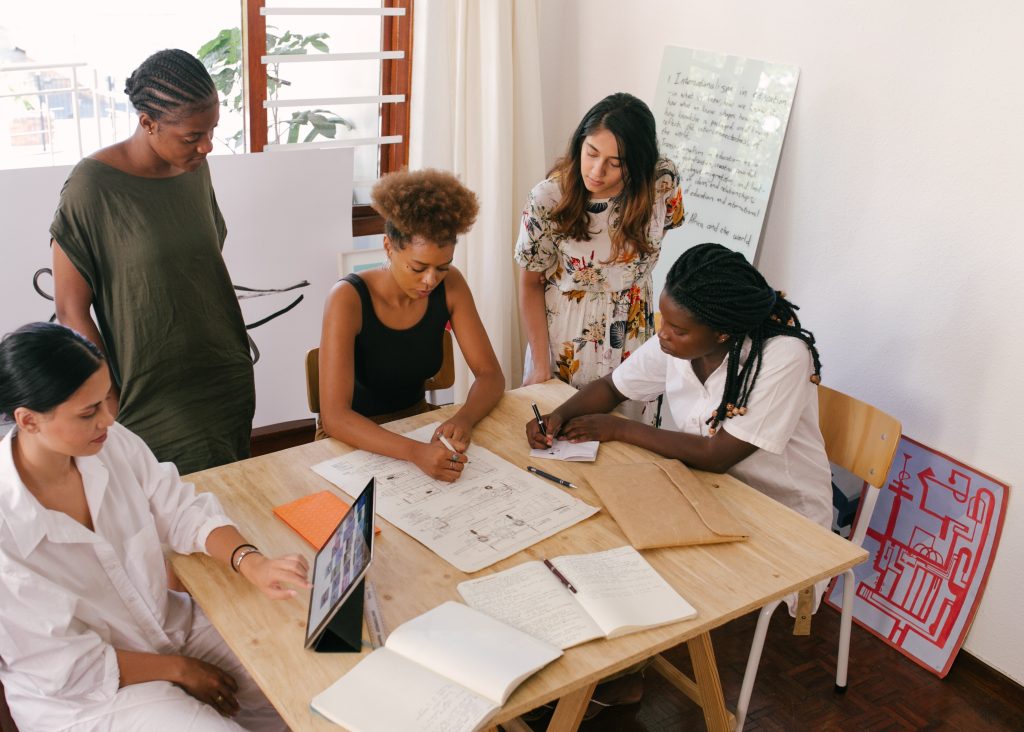
The best tools for data analysis
Data analysis has a vast toolkit. Some leading tools include:
- Excel: A fundamental tool for simple data manipulation and analysis.
- R: A programming language and software environment tailored for statistical analysis.
- Python (with Pandas, NumPy, SciPy): Python, along with its libraries, offers diverse capabilities for data analysis.
- Tableau: An interactive data visualization tool.
- SQL: Essential for data extraction from structured databases.
- Power BI: Microsoft’s suite for business analytics and visualizations.
Salary comparison for data analysts across top countries
Country | Average Annual Data Analyst Salary (2022) |
---|---|
USA | $80,000 |
UK | £45,000 |
Australia | AUD 85,000 |
Canada | CAD 75,000 |
Germany | €50,000 |
Note: The aforementioned figures represent approximate averages for 2022. Variations might arise based on experience, specialization, and other factors.
Conclusion
Data analysis, underpinned by diverse methods and techniques, is pivotal in contemporary decision-making processes. Armed with the right tools and an understanding of prevailing techniques, analysts are better positioned to extract valuable insights from data. Pursuing the Best Data Analytics Certification Programs can provide analysts with advanced skills and knowledge, setting them apart in the competitive field. As the discipline evolves, staying updated and comprehending the intricate landscape of data analysis becomes even more crucial.
FAQ’s
Q: What are the 5 techniques for data analysis?
A: The five prominent techniques for data analysis include:
- Descriptive Analysis: Summarizing the main aspects of data.
- Inferential Analysis: Drawing conclusions from samples about a population.
- Predictive Analysis: Forecasting potential future outcomes.
- Prescriptive Analysis: Recommending actions based on the analysis.
- Exploratory Data Analysis (EDA): Visualizing data to find patterns and relationships.
Q: What is data analysis methods and techniques? A: Data analysis methods and techniques refer to the processes and strategies employed to evaluate and interpret data. Methods are overarching approaches or frameworks used to analyze data, like statistical or qualitative analysis. Techniques are specific procedures or tools within these methods, such as regression analysis or clustering.
Q: Which method is best for data analysis? A: The “best” method for data analysis depends on the nature of the data and the specific objectives of the analysis. For instance, if the goal is to understand patterns and relationships, Exploratory Data Analysis (EDA) might be suitable. Predictive analysis is ideal for forecasting. It’s essential to choose a method that aligns with the research question and data type.
Q: What are the methods used for data analysis?
A: Various methods are used for data analysis, including:
- Quantitative Analysis: Employs statistical and mathematical tools.
- Qualitative Analysis: Focuses on non-numerical data interpretation.
- Comparative Analysis: Compares different datasets or groups.
- Content Analysis: Analyzes textual content.
- Network Analysis: Studies connections in networks. The choice of method depends on the data’s nature and the research’s purpose.
Q: What are the 4 types of data analysis?
A: The four primary types of data analysis are:
- Descriptive: Describes and summarizes data.
- Diagnostic: Delves into data to find the cause of observed outcomes.
- Predictive: Forecasts future trends based on historical data.
- Prescriptive: Suggests actions based on the data analysis.